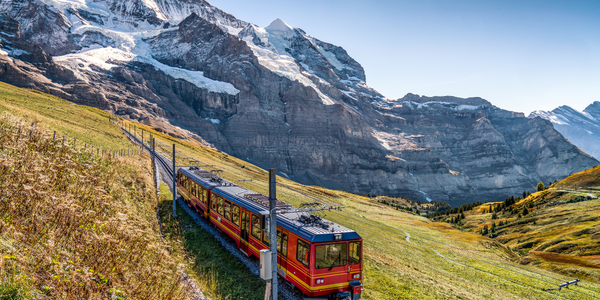
- Analytics & Modeling - Edge Analytics
- Analytics & Modeling - Predictive Analytics
- Functional Applications - Remote Monitoring & Control Systems
- Infrastructure as a Service (IaaS) - Cloud Computing
- Infrastructure as a Service (IaaS)
- Networks & Connectivity - Gateways
- Railway & Metro
- Maintenance
- Predictive Maintenance
Bogies are the most significant components of the rail fleet in terms of lifecycle costs and traffic safety. In addition to creating significant cost savings for the rail fleet owners, data-driven maintenance will enhance safety and the usability of the rolling stock. The predictive maintenance capability will improve reliability of the trains, cost-efficiency and passenger comfort. Train traffic will operate more reliably when it is possible to predict rolling stock malfunctions before they cause disruptions in traffic.
The cooperation is based on VR FleetCare’s technical rail fleet expertise and fleet maintenance optimisation as well as EKE-Electronics’ experience in train automation systems and remote condition monitoring.
The aim is to develop a system that predicts the maintenance need and optimizes the service program of bogies. Data is acquired from the trains by sensors and sensor gateways and a smart combination of cloud and edge computing is applied for the signal analysis. The solution utilises the Adaptive Anomaly Detector and predictive analytics developed by Humaware, EKE’s British subsidiary, integrated into EKE’s cloud based remote monitoring software Smartvision™.
Sensors and sensor gateways will be installed in bogies of VR Group’s locomotives and electric trains during this year. More extensive results of the development work can be expected next year.
Case Study missing?
Start adding your own!
Register with your work email and create a new case study profile for your business.
Related Case Studies.
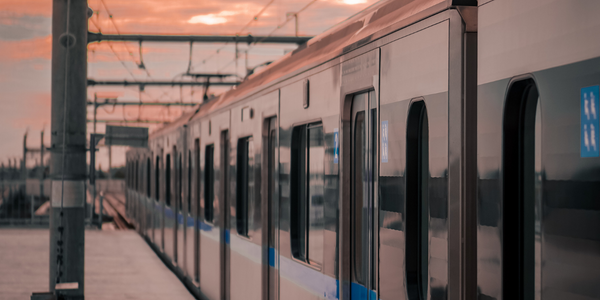
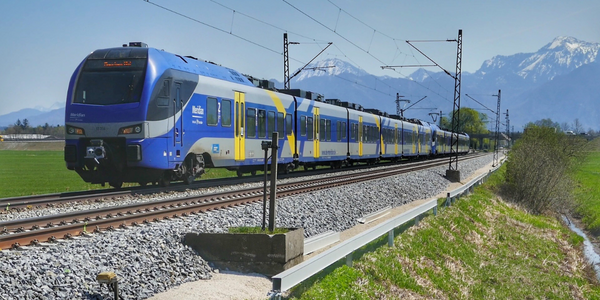
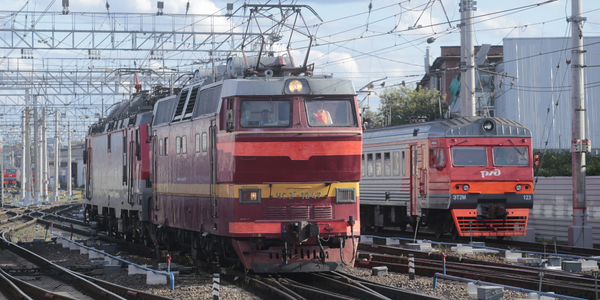
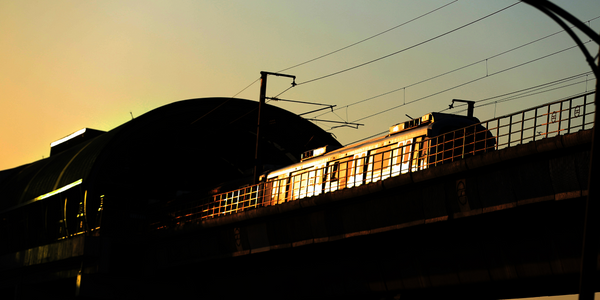
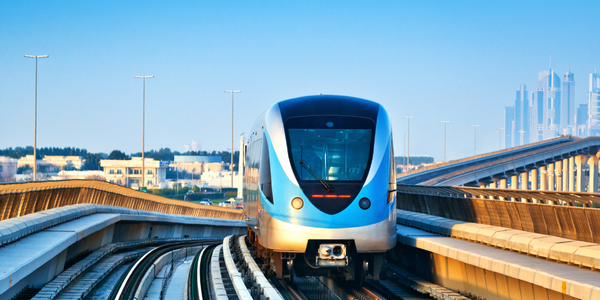