Detecting and Reducing Cost of Fraud Rings
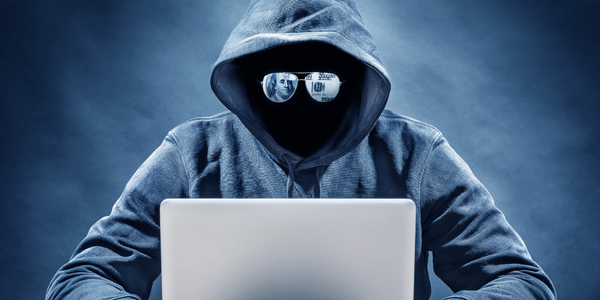
- Application Infrastructure & Middleware - Data Exchange & Integration
- Finance & Insurance
- Business Operation
A leading insurer wanted to reduce its multi-billion dollar expenditures on auto insurance claims, which is its largest annual expense. Currently, the company avoids paying out only about 0.33% of the predicted 10% of fraudulent claims. The insurer uses both manual (60-65%) and automated systems (30-35%) to flag questionable claims. These claims are passed on to the investigative case managers who read adjuster and other member notes, explore the fraud watch list, and search the web. Their existing approach cannot easily discover fraud rings or, more importantly, collusion among other fraud rings. Moreover, the investigative team typically decides in 30 days or less on whether to pay or deny a claim. This is due to heavy caseloads and expectations for a quick turnaround in claim processing in order to keep customers satisfied.
The Natural Intelligence Platform ingested data from three states within the last three years, including auto injury claims (structured data) and MSR notes (unstructured data). Composite memories were created for each claim and similarity analysis was used to provide the insurer insight into data they had not previously seen. Saffron’s associative memory “learns” from legacy claims and new claims; this allowed investigative teams to see patterns and similarities of providers across all claims and easily identify fraud rings. For example, Saffron discovered that a radiology clinic from the investigative watch list was actually part of a much larger fraud ring and a common link that connected three potential fraud rings together. The clinic was previously identified as fraudulent for MRI overcharging, but the investigative team could not view the clinic’s possible connections with other providers. Using Saffron’s “reason by similarity” analysis—where every claim (and associated actors) has a unique, individual signature—Saffron’s Natural Intelligence Platform illuminated all hidden connections to that particular clinic as well as the other colluding rings.
Case Study missing?
Start adding your own!
Register with your work email and create a new case study profile for your business.
Related Case Studies.
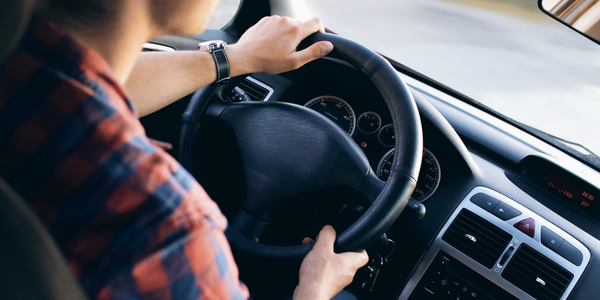
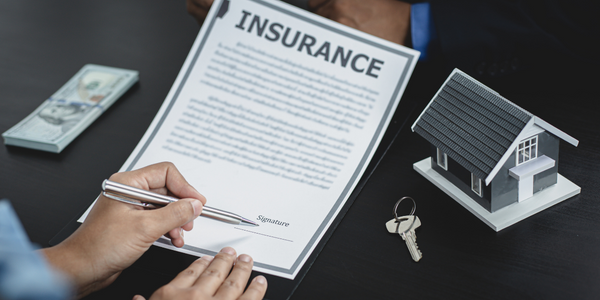
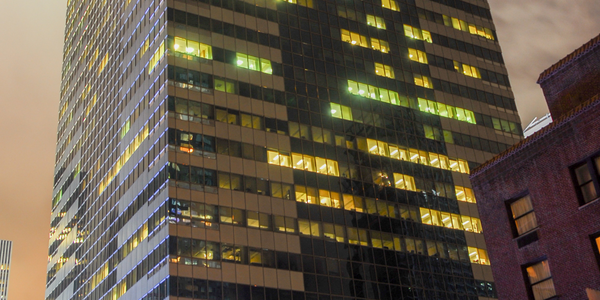
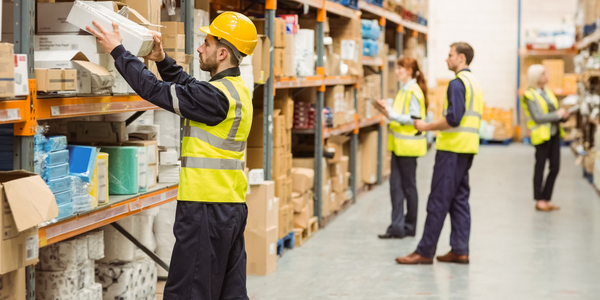
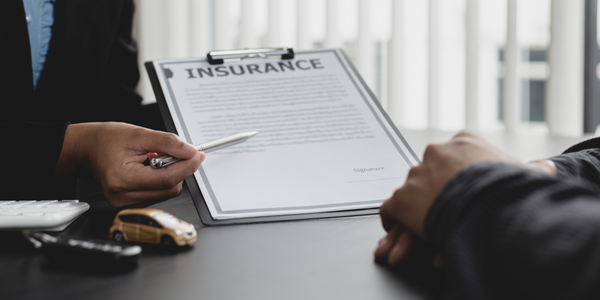
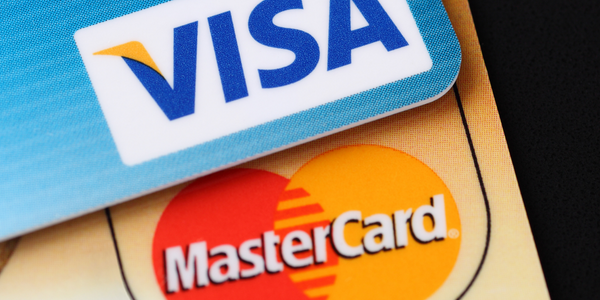